Меню
Шановні колеги! ХІ Міжнародна науково-практична конференція «Інформатика. Культура. Техніка» (25.09.2025 – 26.09.2025) (Детальніше)
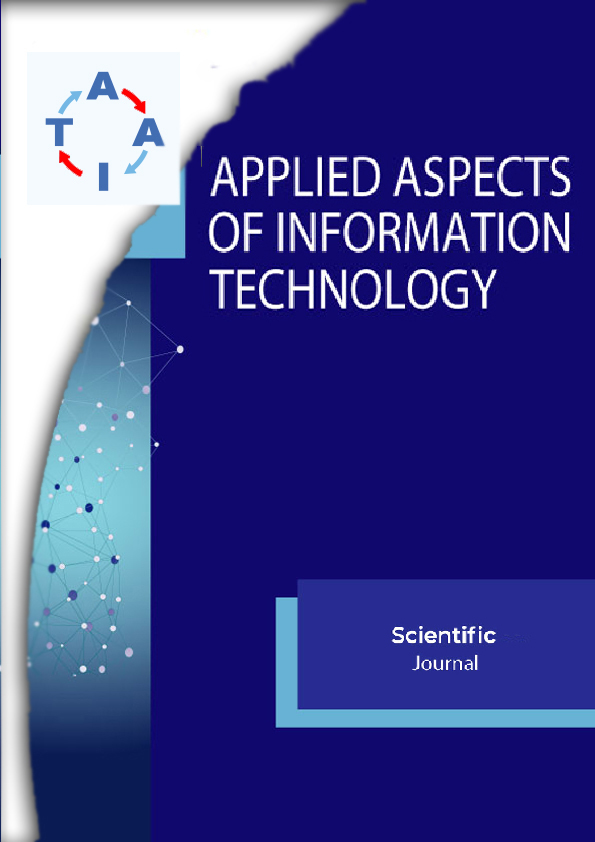
ІНФОРМАЦІЯ ПРО ЖУРНАЛ
Рік заснування: 2018
Галузь знань, яка відображена в самій назві журналу «Прикладні аспекти інформаційних технологій» є невід’ємною та визначальною частиною сучасного життя, яка постійно вдосконалюється. Це сприяє розвитку наукового журналу «Прикладні аспекти інформаційних технологій», основною концепцією якого є оприлюнення теоретичних досліджень та практичних досягнень в галузі інформаційних технологій та забезпечення тісного зв'язку між теорією та практикою. Неухильне дотримання високих вимог до якості рукописів забезпечує журналу «Прикладні аспекти інформаційних технологій» гідне місце серед наукових видань.
Спеціалізація: Технічні науки
Засновник: Національний університет «Одеська політехніка»
Видавець: Інститут комп'ютерних систем
Видавництво: «Наука і техніка»
ISSN 2617-4316 (Print)
ISSN 2663-7723 (Online)
DOI: https://doi.org/10.15276/aait
Ідентифікатор медіа на видання № R30-01945 від 04.12.2023 р.
Періодичність: 4 випуска на рік
Мова публікацій: англійська
УДК 004.9
Журнал «Прикладні аспекти інформаційних технологій» включено до «Переліку наукових фахових видань України» (категорія «Б») з технічних наук. Затверджено наказом Міністерства освіти і науки України №1301 від 15.10.2019, в яких можуть публікуватися результати дисертаційних робіт на здобуття наукових ступенів доктора і кандидата наук (доктора філософії) з технічних спеціальностей (Детальніше список спеціальностей...)
Номери статей з 2018 р. мають DOI від CrossRef.
Електронну версію журналу включено до Національної бібліотеки України імені В. І. Вернадського (НБУВ), Реєстру наукових фахових видань України, загальнодержавної реферативної бази даних «Україніка наукова» та до відповідних тематичних серій українського реферативного журналу «Джерело» (Детальніше...)
Журнал зареєстровано в міжнародних каталогах періодичних видань та базах даних: Research Bible; Academia.edu; Directory of Open Access scholarly Resources (ROAD); Ulrich's Periodicals Directory; Index Copernicus Journals Master List; Google Scholar; Scilit; OUCI; (Детальніше...)
Архіви матеріалів статей зберігаються в бібліотеці і репозитарії eONPUIR та Google Scholar.
Журнал «Прикладні аспекти інформаційних технологій» практикує політику негайного відкритого доступу до опублікованого змісту, підтримуючи принципи вільного поширення наукової інформації та глобального обміну знаннями заради загального суспільного прогресу
Матеріали статей дозволяється використовувати згідно з Ліцензією Creative Commons Зазначення Авторства 4.0 Міжнародна з обов'язковим зазначенням авторства.
На сьогоднішній день редакція журналу не припиняє роботу над розвитком і поліпшенням журналу